Artificial Intelligence and Bias
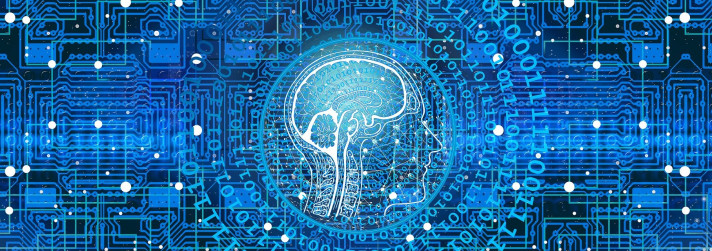
Event Video
It is hard to find a discussion of artificial intelligence these days that does not include concerns about Artificial Intelligence (AI) systems' potential bias against racial minorities and other identity groups. Facial recognition, lending, and bail determinations are just a few of the domains in which this issue arises. Laws are being proposed and even enacted to address these concerns. But is this problem properly understood? If it's real, do we need new laws beyond those anti-discrimination laws that already govern human decision makers, hiring exams, and the like?
Unlike some humans, AI models don't have malevolent biases or an intention to discriminate. Are they superior to human decision-making in that sense? Nonetheless, it is well established that AI systems can have a disparate impact on various identity groups. Because AI learns by detecting correlations and other patterns in a real world dataset, are disparate impacts inevitable, short of requiring AI systems to produce proportionate results? Would prohibiting certain kinds of correlations degrade the accuracy of AI models? For example, in a bail determination system, would an AI model which learns that men are more likely to be repeat offenders produce less accurate results if it were prohibited from taking gender into account?
---
This Zoom event is open to public registration at the above link.
Event Transcript
[Music]
Dean Reuter: Welcome to Teleforum, a podcast of The Federalist Society's practice groups. I’m Dean Reuter, Vice President, General Counsel, and Director of Practice Groups at The Federalist Society. For exclusive access to live recordings of practice group Teleforum calls, become a Federalist Society member today at fedsoc.org.
Evelyn Hildebrand: Welcome to this afternoon's Federalist Society virtual event. Today, May 4th, we discuss Artificial Intelligence and Bias. My name is Evelyn Hildebrand, and I'm an Associate Director of Practice Groups at The Federalist Society.
As always, please note that all expressions of opinion are those of the experts on today's panel.
Today we are fortunate to joined by a very distinguished panel. I will introduce our moderator first followed by our speakers. Our moderator today is Mr. Curt Levey, President of the Committee for Justice, an organization devoted to advancing constitutionally limited government and individual liberty. He serves on The Federalist Society's Civil Rights Practice Group and prior to law school he was involved in AI start up. Mr. Stewart Baker is a partner at the law from Steptoe & Johnson in Washington, DC. He's the General Counsel of the National Security Agency, and he's the host of the Cyberlaw podcast which is now in its 360th episode. Nicholas Weaver is an International and Computer Science Institute and Lecturer at UC Berkeley. His primary research focuses on network security, notably worms, botnets, and other internet scale attacks and network measurement. He now spends a fair amount of time translating technical issues into understandable material for policy makers.
After our speakers give their opening remarks, we will turn to you, the audience, for questions, so be thinking of those as we go along and have them in mind for when we get to that portion of the event. If you would like to ask a question, please submit those questions via chat, and our moderator will read them out and hand those over to our speakers.
With that, thank you for being with us today. Mr. Levey, the floor is yours.
Curt Levey: Thank you, Evelyn. This is a subject of great interest to me given my career which has involved both law, including discrimination, and being in the AI field. I'm clearly not alone in this being of great interest because most discussions about AI these days mention the potential for AI bias against racial minorities and other identity groups. And just a few of the many areas where bias can creep in is facial recognition, lending, bail determinations.
I'd like our panelists to discuss whether potential AI bias is indeed the grave problem you would think from the debate, or is it exaggerated or misunderstood. And then also answer the related question of AI, despite its flaws, is it an improvement over human decision making which has been known to have an irrational bias or two. AI models may not have traditional prejudices, but they learn by discovering patterns in real world data, and they may discover correlations that have a disparate impact on one group or another.
In any case, legislation is being proposed at the state and federal level and even enacted to address these concerns. I've heard the Biden administration is working on agency regulations on AI and bias. So I'd also like our panels to discuss the extent to which we need new laws beyond current discrimination laws that already give human decision makers as well as the tools they use that already govern people, basically. So without further ado, Nick, can you start us off?
Nicholas Weaver: Yeah. I will start off with basically a discussion of what people call AI today. And it's really a process known as machine learning. And this is actually a very old thought. People have been looking at machine learning since the '90s if not earlier. It's just that in the past few years, we've had a revolution in the capability of machine learning, not because the underlying algorithms have gotten better but because of a quirk of happenstance. And that is that the underlying problem behind machine learning, what we call dense matrix multiply -- take a whole bunch of numbers, multiply them together to get a whole bunch of numbers -- is something that works really well on graphics cards for some strange reason, well, not strange reason, but a coincidental reason. So as a consequence, we've had a revolutionary leap in the amount of compute we can throw at the problem.
So how machine learning works is you have a whole bunch of data. This data has patterns, but you don't know what the patterns are and don't know the meaning of the patterns. So you create a whole bunch of labeled data. So let's take an example of I want to do dogs and wolves. I take a whole bunch of photos of dogs, whole bunch of photos of wolves, download them off the internet, and basically split them out into a set of training data that is munched on in an attempt to find the underlying pattern. And then I verify it by taking some of the data I didn't use, and I test it, and, golly gee, my photo recognizers quite good at telling the dogs from wolves because it picked up on the trees and snow in the wolf photos, not something innately wolfness. And in fact when you dig into more details, you see that, for some reason, the sled dogs photos tend to get misclassified more than the other photos.
So here we get into the two big problems of machine learning. One, what are the biases in the training set? And two, how do you know what the machine learner actually distinguished on? Because when you can come up with a distinguisher that you can explain, you don't actually end up needing the machine learning any more except to hunt for the distinguisher. So for the dogs and wolves example, it's quite subtle for wolf-like dogs. What you have to do is look at the eyebrows. Wolves are a bit bigger. Wolves do not have expressive eyebrows. But it's actually a really hard problem to distinguish dogs from wolve-like dogs because they're so genetically similar, they'll actually interbreed, so the question is is machine learning the right answer in the first place versus just nailing them and do a DNA test?
So those are the problems. We don't know what goes into the training set, and we don't know what patterns we are getting. And then it gets used by companies that want it as a black box. So they view the training data as a competitive advantage. They view the deniability as a competitive advantage. So like Facebook uses a whole bunch of machine learning in their ad profiling of people, and it's not their fault that alcohol ads get presented to those under 21. It's not like Facebook knows their birthdates. It's just coincidental. So we have that go on as well.
It's easy to misuse. Every couple of years we get a paper recapitulating phrenology. For the trivia buffs, that's the notion that the bumps on your head somehow indicate aspects about you. The only thing that phrenology really indicates is that the people involved need retrophrenology, which is you beat them over the head until they understand what they're doing is wrong.
The classic was of doing it is you take a bunch of photos of criminals and a bunch of photos of non-criminals, and you come up with a distinguisher between the two. But how do you get the photos? How do you know that you aren't putting bias into the data set? For example, one thing that you get is it's clear that our policing has biases in it. So any data that captures the results of policing decisions is going to have that bias built into it unless you're very, very careful, and even when you're done, the question is what are you distinguishing on. This keeps cropping up over and over again.
So every time Silicon Valley does a face recognizer, it seems to be vastly worst on African American faces, and they are unable to answer the question is it that your training data is not as good, or could it be fundamentally harder because things like freckles and skin moles have a lower contrast, and so unless your camera's good, you don't see those. They have no way of answering the question of why, but you start to think it's biases in training set when Asian faces seem to do vastly better on Chinese built image recognition systems than they do on U.S. built image recognition systems.
These creep in all the time, but in terms of legislation, I'm not sure if legislation is necessary beyond having systems be able to explain what they're decision making is because then you know if there's bias involved or not.
Curt Levey: I guess we should hand it over to Stewart at this point.
Stewart Baker: Okay. That was very interesting, and I do want to pick up on a few things. I'll start with explainability, which the AI experts tell us is a real problem, that it's hard to get AI to explain itself. That is certainly the experience of most people in the field. But what we do when we don't get an explanation in this area is we anthropomorphize the AI. In fact, I would say we misanthropomorphize the AI, if that's a word. We assume the worst about the intentions of artificial intelligence which we have imbued with a kind of personality. And so there is a tendency -- it's either a natural human tendency or it's a liberal bias on the part of the investigators and the journalists that cover the research -- to say if there is any adverse impact on any of the minority groups that I care about, then I'm going to attribute that to racism, sexism, trans phobia, whatever, on the part of this anthropomorphized engine.
That strikes me as the first problem in making policy in this area is that there is an enthusiasm for finding bias that vastly exceeds the amount of bias that it's fair to identify. I think Curt talked a little bit about whether there's bias in determinations about sentences and parole. That's a pretty good example. Everybody's heard this, those studies in the field that Broward County was using a racist mechanism for determining who was going to be a recidivist. It turns out that you could say that about any use of the AI that Broward County had engaged in. They had an algorithm that was equally predictive as to white and black inmates. It was almost exactly the same mistake.
So ProPublica when down there and said well, let's take a look at after the decision is made, when we can see whether they actually were recidivists and see what kind of error rate there was. They discovered that there were a lot more errors that kept people in jail if they were black and a lot more errors that let people go if they were white, and they said obviously this is racist. And we all believe it from the press coverage.
But the folks that looked at this and said well, why don't we try to fix that soon realized you can't fix that without wrecking the fairness in the predictive quality of the AI. It's just mathematically not possible in a context where recidivism rates are different between blacks and whites to have fairness both in the forward looking prediction and in the backward looking determination of whether things were done properly, predictions were right or wrong. So it was heads, ProPublica wins; tails, Compass loses. They could always find bias. I think we need to be careful with all of these studies to ask were there alternative calculations or explanations to the immediate assumption of bias, which, in especially academia, is always the first resort of the researchers.
I think Nick's quite right about face recognition. There's a perfectly -- face recognition is less good on dark faces, less good on female faces, less good on young faces. And when you think about it, if you believe that face recognition is mostly looking for bone structure, if there's less contrast because the shadows are harder to see on dark faces than white faces, if it's harder to see the bones because there's more subcutaneous fat on young faces or female faces than old, male faces, you're going have a much easier time finding the bone structure of old, pale men to my horror. I find that every morning when I shave. That's an understandable explanation. No one has really spent a lot of time asking that because it serves ideological purposes to say oh well, it's because of racism in the design or the training of the algorithm.
I worry that we can quickly get to an accusation of a bias that is not grounded in any serious consideration or alternatives and that that drives us down a cliff that we will really regret because the next step is to say well, then we need to demand fairness of every algorithm. And the researchers have been quite candid in this areas of saying well, sure we can give you fairness. We can give you 20 different kinds of fairness. You just tell us which fairness you want. You want to have all groups that you care about not disadvantaged by this? We can set it up. We can just shim the data, rig the data so that it refuses to find distinctions among those groups. Or if you want to find pure accuracy and don't care and you think that's the fairest outcome, we can solve for accuracy. Or we can solve for individual fairness of the sort that Martin Luther King's "I Have a Dream" speech called for, content of character and not color of skin.
If you leave the question of what is fairness up to the designers of the algorithm and the people who are on the front lines of most of the decision making here, the Office of Civil Rights at the Justice Department, especially in a democratic administration, you're going to get group rights. You're going to get a determination that every group should be protected against disparate impact and not just every group, but every intersection of groups. That requires a lot of shimming of the data to achieve, but once you've achieved it, and people are doing that now much more often with synthetic data. They're just making up data -- a lot of good reasons to make up data, but if you make up the data or if you run a machine learning system in which you say to the machine go through and tell me what percentage of each group you've promoted as relevant and treat it is a positive, and if you produce a result that is not within 10 or 20 points of being proportionate to whatever representation we're going to put in front of the machine, we will reject it. We'll tell the machine it got it wrong until it learns -- it's sort of like a admissions officer at Harvard or somebody operating under a consent decree that sets goals but not quotas -- that there are right answers and there are wrong answers, and you might not want to talk exactly about how you got to the right answer, but you, by god, will get to the right answer. And you're essentially building a set of quotas into every definition of fairness you apply.
That takes me to the last point which is that we have used quotas like nitroglycerin in policy making. It's very, very strong medicine, and it has the capability of being enormously socially divisive as witness Harvard admissions. Having all of those quota systems built into every decision that is touched by AI is a dramatic expansion of how we understand decisions to be made and, I think, in the long run, imposes a kind of AI fairness tax because it says no matter what the reasons for the statistical disparity you encounter may be—there may be a dozen environmental factors that you can try to trace back if you choose to systemic racism, but are none the less very real and have a very real impact on these individuals—you have to ignore all that in the interest of producing a proportional representation of whatever group has been chosen for this in the reward that is being handed out. It almost becomes a weird reparations program in which all of those past problems are imposed as something that has to be solved by whoever is using the AI for this particular decision.
It's very likely to produce disparities that we don't like, and maybe more important and this is my last point, it buries the debate deep in the mysteries of artificial intelligence. The people making the decision -- and Nick said this about some of the advertising decisions -- the people making the decisions can say, "I just want to use artificial intelligence, but of course I want it to be fair." Then they say to the people giving them the algorithm "Is it fair?" And they say, "Yes, we took it to experts who come from academia and are determined to root out the unfairness, and so this is a fair algorithm. Don't ask any more questions."
And if course the decision maker is happy to get the results that they get, so they don't ask any questions. Nobody knows how much or how little discrimination actually ended up in the algorithm because it's hidden behind a veil of unexplainability. I just think that's the wrong direction for us to go. From a legislative point of view, we probably should stop talking about fairness and start saying we need to know every time you rig these results, tweak these results, use synthetic data for these results in order to achieve some definition of fairness, and we want to know exactly what your definition of fairness is.
Curt Levey: Thank you, Stewart. I'll throw a few questions at you and Nick, and then we'll turn to audience questions. Let me direct this first one at Nick. What do you say about Stewart's point which is that let's say you pick good data that accurately reflects the world and you carefully pick your variables and you minimize error or put it another way, you maximize the accuracy of the system, and still, the system is recommending more negative bail determinations for black people than for white people. Do you just accept that after carefully studying it to make sure that it wasn't bad data or bad variables? Or do you need to fix it despite the fact that it would increase the error made by the system?
Nicholas Weaver: Well, it depends. Are we having single-sided error or are errors for one group different from another? What is the underlying rationale for the decision making? This gets back to the explainability problem that the algorithm designers aren't really designing algorithms. They're curating training data into a black box that is designed to give them an answer that they cannot come up with a rationale for otherwise. Because if they can, they don't need the machine learning. So that's the fundamental problem is people are using this in a way that is deliberately obscuring the problems, and then other people go along and go hey you have a problem. And then we get Stewart freaking out about the woke mob when he just made a very convincing argument that because there is underlying disparity in the underlying real world data, what do we do about that?
Stewart, I never thought you'd be quite so progressive [laughter 00:24:43].
Stewart Baker: I'll try to overcome it.
Nicholas Weaver: The other problem is is there is enough evidence out there that there is a tendency to like that machine learning can launder stuff and can find confounding variables very easily. So say your training data explicitly excludes race but includes zip code of residence. That's going to be a very strong proxy. If it also includes name as well as zip code of residence, that is going to be a really strong proxy. And the machine learner is going to instantly figure out the proxies for the thing you don't want to officially select on and select on that.
Curt Levey: Yes, but it's not going to do it out of malevolence. It's going to do it out of the fact that again, those "proxies" allow it to minimize error. So let me ask you this question. Nobody's saying that AI systems are perfect, but humans are not perfect either. Humans do have malevolent biases and sometimes intend to discriminate, and they often know your race, gender, sexual orientation, whereas you can hide that from a machine. So let me ask you both to do sort of a comparative analysis. Assuming flaws in the AI systems, are they still better bias-wise than us deeply flawed humans?
Nicholas Weaver: It depends. I know you hate that answer, but it really depends on the context of the system and who's making the decision and whether the decision makers themselves are aware of their own biases. That actually makes a difference that if people are aware of their unconscious biases, the biases become less.
Stewart Baker: My sense is, yes, this is powerful technology for finding weak variables and maybe a lot of them. And those weak variables when combined are likely to add to the accuracy of the decision. What we do as human beings is if we have four variables in mind when we make a decision about how good somebody is at a particular thing, we're probably at the limits of our analysis. It's very easy, all the talk about unconscious bias, builds off of the idea that we take short cuts and sometimes race or ethnicity or gender is a pretty good short cut for determining people's ability in the long jump.
Nicholas Weaver: Or it turns out that when you actually study the effect, that bias turns out to be wrong. So if you look at studies of corporate leadership, women are actually better at that. So the biases that have caused men to get promoted over women in those circumstances has often been self-defeating, but until you really get at it, how do you know?
Stewart Baker: And only AI, well, not only AI, but AI is a way of finding that in contexts where you can determine with some specificity what is success and what is failure and compare it to a very large data set. So I'd say AI probably deserves the benefit of the doubt over the human guesses. That doesn't mean it's perfect. It's got the wolf problem for sure. I think we always need to worry about that, but when somebody comes to bring you a bias story, they need something other than just to say oh well, there's probably bias here in the decisions that were arrived at by the people in the training data. I think that's a cop out.
Curt Levey: Let me throw a similar question out, too, on explanation that's long been recognized as a weakness in AI systems is their ability to explain their reasons. It goes back, well, certainly for as long as I've been involved in the field. But again, humans have their limitations there too. They rely a lot on intuition, and sometimes they're not truthful about why they made a particular decision. So I'll ask you again both to compare and contrast the relative weaknesses and strengths of AI versus humans.
Nicholas Weaver: I think that human decision makers are far better at explaining their rationale. Now some of it may be self-justifying. Some of it we have the self-justification problem. We have the problem of basically people laundering their biases in justification, etc. But at least they can try. The biggest problem I have with applying AI to people is that it can't show its work. It literally cannot. It is an open research problem to get the AI to show its work. If you can get it to show its work, it doesn't need to be AI.
Stewart Baker: I'm not sure that that's -- I hesitate to challenge Nick on the technology, but from the point of view of explainability, there are things that one can easily imagine, techniques for extracting what were the significant factors and trying to give weights to those, varying the data inputs in ways that tell you want those variations meant in terms of outcomes that might give you a pretty good idea of what's happening inside the AI. And I do think that if you can do that, you're likely to discover something. You're likely to discover -- I don't know -- that left-handed people have an affinity for certain kinds of mechanical work that no one imagined because it was too mild a variable to become a stereotype. So I think explainability may allow us to take AI insights and actually use them, and we wouldn't have those insights without the AI having done the analysis.
Curt Levey: Thank you. Let me ask you another question. The very purpose of decision making models, AI or otherwise, is discriminate, for example, discriminate between people who are good and bad credit risks as you're building a lending model. How do we tell the difference between useful discrimination and bad discrimination? Sometimes, it's easy. I think we would all agree a rational racial bias is wrong, but sometimes it's not so clear cut. What if it discriminates based on the neighborhood you live in? How do we determine what's okay and what's not?
Nicholas Weaver: So this is a hard problem wherever that whatever the decision maker is, that's a hard problem. And that's a greater societal problem. Poor neighborhoods are worse credit. Poor neighborhoods have a long racial history behind them. Are you justified in using zip code to make decisions on home lending? And the answer is no, not directly because this was used for explicit discriminatory purposes in the past. That's the anti-redlining laws.
Stewart Baker: I think that's a good summary of how we have approached these issues in the past but may not be if we're going down the path we're going with AI. That is to say, the presumption has been that using certain categories, race, ethnicity, gender is just not right. You should not use those as shorthand for people's capabilities because of history, and we have a relatively small list of those, although it's become a little bit of an opportunity to add group after group that are in status, marriage status, etc. But on things like ethnicity, race and gender, the history of discrimination is such that we just say don't use them.
Beyond that, there are any number of things that correlate very strongly with all of those things, and we have been quite slow to say oh, you can't use anything that correlates with those. There has to be some history and usually some sense that the correlating item is being used deliberately by lenders, say, redlining neighborhoods because they're black, and they don't want to lend to blacks. So it's easy to say I don't want to lend to anybody in that neighborhood.
If you try to take that approach and generalize it to say we won't allow anything that can later be shown to correlate to a particular characteristic and say you can never use any of those, you are imposing quotas on every decision that falls within AI's purview.
Nicholas Weaver: This is one of the real hard problems is because AI is so easy to misuse and find these hidden cofounding variables. I have a quip: machine learning is a great way to teach a computer to be a racist asshole. And my worry is that some people like it that way.
Curt Levey: You're talking about discovering variables that you might not otherwise discover. Let me throw a couple of examples at you and ask you what you think. Say we have a model for bail determination or parole, and it finds that being male makes you more likely to be a repeat offender. And this it improves its performance, minimizes its error by taking gender into account. So you have it's definitely judging people by their gender to some degree. Is that okay?
Nicholas Weaver: That is the $64,000 question. This is also where explainability is absolutely essential if we want this for real world decision making is because if you can say the AI is making this decision because X. Then you can go is that actually a fundamental decision? Is it the right decision? And that's very, very important, and until we get explainability, that's my worry is that we can be baking in these biases from the data, from this curation of the data, from signals that you don't even realize are in the data. So we're stuck with looking at outcomes.
Stewart Baker: Yeah, let me pick up on one aspect of that which is ProPublica, I’m sure, found that that's exactly the case that women are less likely to be recidivists, and the artificial intelligence did predict fewer women would be recidivists. And they didn't make much of it. I think that's partly the misanthropomorphism that I talked about. They said, well, if they're discriminating in favor of women, they can't be sexist, so I guess it's not sexism. It's partly very practical. I think if you shim the data so that groups that have traditionally been discriminated against do better, nobody's going to complain. It's going to sound like fairness, or very few people are going to complain until they're disadvantaged by it. If you shim the data to make it harder for women to get bail, you're going to get a sex discrimination lawsuit because you deliberately introduced a factor that's not justified by the data to equalize the results. I suspect that people don't do that because they have a sense of who's supposed to be protected by Civil Rights laws, and it doesn't include the majority.
Nicholas Weaver: I think it's more subtle than that. The Broward County case, some of the flagship decisions are nearly identical crime, vastly different criminal history. The white dude with the significantly worse criminal history given much lower bail than the black dude without the criminal history. This says that the machine learning was picking up on something outside what an explainable system would do and that when you go back and do a much simpler machine learning model based on just recidivism rate based on prior criminal history, you actually get better results.
Stewart Baker: Especially if you throw in age, it turns out you don't need the AI at all.
Nicholas Weaver: Yeah. This is an example of where explanation defeats the AI. The AI was making wrong decisions, and once you explain how you look at the decision making, you come up with a much simpler criteria that's not only much more effective but eliminates all the biases outside that caused by the underlying policing.
Stewart Baker: Another tough example was alluded to earlier when we said that Asian facial recognition systems are typically better at recognizing Asian faces. As a matter of fact, I'm aware of at least some studies that showed that systems in East Asia did worse at recognizing Caucasian faces than the faces of East Asians. I guess you could say that's discrimination against Caucasians. Now, is that a problem, or is it the right result given that this model is going to be used primarily on East Asian faces and you want to maximize overall error?
Nicholas Weaver: It really depends on context. Face recognition, truth be told, the bias in that doesn't bug me as much because what really bugs me is actually how it's being used. Why are the Chinese facial recognition programs so much better at Asian faces is they're basically trying to conduct mass surveillance in Xinjiang.
Stewart Baker: That would become even better at Uyghur faces, huh?
Nicholas Weaver: Yeah. And that's what their optimizing for is their particular mission. And face recognition seems to be a hot button on the AI field, and it's not the one I worry about because you get the occasional false positives, but you go back and fix those, compared with the problem of decision making about people is a much bigger problem.
Stewart Baker: I think the East Asian facial recognition being better on Asian faces example probably is an example of the first order of bias correction that everyone should look for. Rather than saying oh, another racist algorithm, you might say how much training data with respect to particular groups does this algorithm have. Obviously, there's a lot more Asian faces available to do facial recognition on in China or Korea or Japan than in the United States. So you'd expect subtle differences to be picked up on more easily by algorithms that have had more training data.
So before we jump to bias, we ought to ask is this particular group that we think is suffering from bias simply too small, too underrepresented in the training data for us to have confidence in the outcomes. And that's probably the case with a lot of the facial recognition problems. It's probably not perfect to even if you did that because there are going to be, as I've said, questions about shadows that don't show up on darker faces. But I think the first thing we ought to ask is is there a way to do use more data to get better results.
Nicholas Weaver: Or make the training data actually available for examination. Congratulations, Stewart. How do we do that? Regulation.
Stewart Baker: Okay. I would rather that we were asking the question how have we improved the accuracy than how can we ignore accuracy and stick in a shim to achieve the social goal that we think is appropriate.
Nicholas Weaver: And in order to understand that, you're going to need regulations that make training set datas available for examination and explainability.
Stewart Baker: Yeah, that may well be. Here's the other response to the problem of face recognition data, and it cuts against Nick's overall rule which is maybe you don't need the AI at all. But with face recognition -- maybe it's because humans are generally pretty good at it -- the answer in most cases is to say use it to narrow your suspects, but don't arrest people on the basis of the machine told me to. You ought to hold the police responsible for looking at two people and saying it’s the same person as opposed to say I don't know but the machine said it was.
Nicholas Weaver: Agreed. And the other things is is you need to understand that when you're doing small recognition which is what you're having the police do, your error rate is less of a problem. So if I'm comparing two photos and my error rate is 1 percent, I'm going to be right 99 percent of the time. If I'm comparing one photo against a database of 10 million and I have a 1 percent error rate, I'm going to be getting 10,000 errors for the one right answer.
Stewart Baker: But if you're a cop and you're looking through a list of suspects, you'd rather look at 10,000 than a million.
Nicholas Weaver: Right. And also what you do is you basically do take advantage of separate errors in a different problem, and I agree that this is an example of what should be good AI regulation. Any arrest based on facial recognition must be confirmed by the officer before arrest.
Stewart Baker: Yeah. That makes sense to me.
Curt Levey: All right. Let's take some audience questions in the last 10 minutes or so. One caller asks, "How do we know that there's not factors other than bias that are resulting in the disparate impact, and how would we empirically test the hypothesis that it's neutral rather than discriminatory factors?"
Stewart Baker: I think the first step -- I would offer just a first step is you ought to ask are there alternative hypotheses, and can we test the alternative hypotheses. Frankly, I understand that there's a body of thought in the country that says no, no, it's a racist country. Systemic racism, that's the first explanation and the easiest explanation, and you've got to rebut it before I'm going to listen to anything else. But I just don't think that's right. I think it's fair to start with the presumption that there may well be an alternative explanation and that it's at least as good at shouting systemic racism.
Curt Levey: Any thoughts, Nick?
Nicholas Weaver: The thing is is until you try for explainability, you're not going to be able to do this one way or the other. The point of regulation that I think is necessary is to require at least some degree of explainability when you make a decision concerning a person. That we have narrow areas where that happens and that keeps things a lot more honest. So like on credit decisions, if you're denied credit, you get the right to the underlying data that was used to make the decision and can therefore look for errors and stuff like that. If we're going to think about how to deal with these problems, that, I think, is the best way to start going about it is regulation that allows or that mandates at least an attempt at explainability.
Curt Levey: Well, we actually have a question about explainability. And it's, "Over time, with greater scientific sophistication, will the day come when explainability is no longer a problem, when AI can easily explain itself?"
Nicholas Weaver: I would love this. This is an area that is a lot of focus of research. And I, for example, have colleagues that are in this and have discovered such things that it turns out that your neural networks are actually really crappy memories. There actually isn't all the much true information that's being learned. Better explanations will really help mitigate a lot of these problems because it will allow you to determine whether it's bias that was baked into the training set, bias that reflects the biases in our society, or just bad luck. And being able to do that is an active area of research, and I really hope that progress is made because it really reduces the disruptive nature of this debate because there's no longer a debate of is the AI biased, it's just you ask it what it did.
Stewart Baker: I am reminded -- I'm pretty sure this is what was said by a researcher into human consciousness who said talking about all of the research that suggests that we do a lot of things before we decide to do them and then later tell ourselves that we decided them, that our consciousness is really just a PR agent for the rest of our decision making processes which are substantially less elegant or attractive. So human explainability is probably not to be -- shouldn't be our model because I think you could easily discover and find a way to design an explainability algorithm that was as afraid of admitting to racism as the average American --
Nicholas Weaver: - But the average American is a lot more capable of justifying their decision making, so --
Stewart Baker: - Yes. I think what's [crosstalk 00:50:19]
Nicholas Weaver: - I'll be happy if AI explainability is a good and as biased as you are, Stewart.
Stewart Baker: Fine. I do think you could end up with something that is basically casting about for alternative explanations and rolling them out until they've all been shot down and say oh, I've guess I've got nothing else to explain it. And maybe that's where we end up.
Curt Levey: Well, speaking of humans versus machines, this speaker points out that "Both speakers operate from the view that partially subjective decisions should be automated so bureaucrats and judges can impose decisions without having to think about them. Is that problematic?"
Nicholas Weaver: I think that is problematic, and I don't mean to say I'm necessarily in favor of machinery decision making. Until the machinery decision making can explain what it's doing, you have real worries. I really don't like this trend towards AI automate the decision making involving people except in cases where you can show the work or you can involve a human in the loop so that the human is able to check the AI or vice versa you have the AI check the humans.
Stewart Baker: I want to argue with the assumption of the question too. I’m not sure that any of us is saying the best thing would be to get those humans out and just let the machines decide it. I think anybody who's been on the receiving end of algorithmic decisions knows that they suck and that one of the increasing luxuries that money buys you and status buys you in this country is escaping algorithmic judgment and getting a human in its place. Those of us who manage to skip the line or endure the line so that we're no longer dealing with the text messages from a machine know exactly what that's about. But putting a human in the loop just means we're going back to the old and flawed mechanism for making decisions, and we may not love that either.
Curt Levey: Let's wrap it up with a question I posed in the intro. AI bias can potentially be addressed with the same antidiscrimination laws that govern human decision makers and the tools they use such as hiring exams are often the subject of discrimination legislation. So do we need new laws? Or maybe I'll put it another way. If I use hiring tools that result in discrimination, should it matter whether it’s a dumb employment test or a sophisticated AI tool?
Nicholas Weaver: I'll say no. It shouldn’t matter.
Stewart Baker: I think it's harder to say the AI tool was not related to the job classification or the job qualifications because it is based on people who have succeeded in the job in the past. What else are you going to use to determine what qualifications are? The alternative is to say any tool that doesn't produce the kind of numbers that we demand is going to be struck down as discriminatory. I do think that's where we're going if we don't stop and think about what AI fairness actually means. But I don't think that's the place we want to end up.
Curt Levey: So do I hear you both saying that, for now, we probably can deal with this through existing laws? That doesn't mean deal with it well, but that really these problems exist with or without AI.
Nicholas Weaver: I'd say these problems exist with or without AI. It's just we should resist the AI defenders who use the algorithm as an excuse.
Stewart Baker: Yeah. I worry that the fairness model, the use of fairness as a slogan, which really means proportional representation in all things governed by AI, is, without some further action by legislators, going to sweep a whole bunch of decisions into what amounts to a quota system.
Curt Levey: All right. Well, we'll have to wrap it up. I thought it was a very, very interesting panel. Thanks to two great guests. So thank you, Stewart, and thank you, Nick. And we're done for today.
Stewart Baker: Thanks, Curt.
Curt Levey: Thank you.
Evelyn Hildebrand: And on behalf of The Federalist Society, I want to thank our experts for the benefit of their valuable time and expertise today. I want to thank to our audience for participating and sending in your questions. We welcome listener feedback by email at [email protected]. Thank you all for joining us today. We are adjourned.
[Music]
Dean Reuter: Thank you for listening to this episode of Teleforum, a podcast of The Federalist Society’s Practice Groups. For more information about The Federalist Society, the practice groups, and to become a Federalist Society member, please visit our website at www.fedsoc.org.